Fewer Train Service Disruptions and Operational Delays:
Shifting from Cyclic Maintenance to Condition-based Maintenance
Smooth and uninterrupted railway operations rely heavily on the maintenance of rail vehicles, components, and infrastructure. Traditionally, a cyclic maintenance approach has been employed. However, this approach poses challenges. If maintenance intervals are too short, it results in excessive maintenance costs, while longer intervals can lead to costly disruptions and failures.
Optimized maintenance intervals enable uninterrupted operations with lower maintenance costs
Determining the need for maintenance and upkeep measures is not solely dependent on elapsed time. Numerous factors, such as
utilization and load,
environmental and operational influences
age of components
influence the wear and tear of rail vehicles, individual components, or infrastructure. These factors are typically measurable. Our core competency lies in monitoring, with a focus on vibration and acoustic data in conjunction with operational data and integrating them into monitoring systems. For this purpose, algorithms are developed based on mechanical models, enhanced by AI components. The objective is to identify precise condition indicators and key performance indicators (KPIs). With these insights, infrastructure and components can be continuously monitored and maintained based on their condition.
![[Translate to English:] Rail Anomaly Indicator 2019 [Translate to English:] Rail Anomaly Indicator 2019](/fileadmin/dienstleistungen/rail/bilder/rail-anomaly-indicator-2019.jpg)
Predictive Maintenance is a broad term that has been discussed in many industries for quite some time. The benefits of this concept for the rail sector are evident and widely acknowledged:
Manufacturers and operators
of rail infrastructure and
infrastructure components
![[Translate to English:] Schineninfrastruktur [Translate to English:] Schineninfrastruktur](/fileadmin/zentrale-elemente/icons/rail.png)
- Optimized monitoring of wear condition
- Improved maintenance planning and management
- More efficient utilization of existing personnel resources
- Optimized material usage
Rail
vehicle
operators
- Enhanced safety and reliability in train operations
- Reduced delays and cancellations
- High fleet availability and reduced need for replacement vehicles
Manufacturers
of rail vehicles
and vehicle components
![[Translate to English:] Hersteller von Schienenfahrzeugen und Fahrzeugkomponenten [Translate to English:] Hersteller von Schienenfahrzeugen und Fahrzeugkomponenten](/fileadmin/zentrale-elemente/icons/control.png)
- Identification of weak spots to optimize future products
- Improved vehicle availability and reliability
- Increased customer satisfaction
Achieving predictive maintenance in three steps: your customized approach
For many, the real challenge lies in implementation. What data should we gather? And, more importantly, how can we extract concrete value from this data?
Our three-step approach makes integrating predictive maintenance into your existing system far more straightforward than you might anticipate. Explore each step by hovering your cursor over it to gain deeper insights:
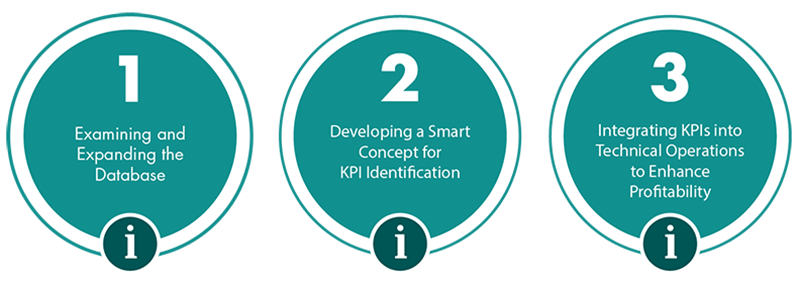
Our service portfolio in the field of maintenance and repair in rail transportation
- Optimization of maintenance intervals
- Monitoring of critical infrastructure components (rail, switch, track alignment)
- Evaluation of operational stress factors (shock monitoring, dynamic loads)
- Monitoring of maintenance-relevant components (wheelset, bogie, wheel bearings)
- Early detection of defects and anomalies
- Load detection (service life, validation, design loads)
WHAT MAKES US EXPERTS?
Please contact us personally
________
We will be happy to advise you on multibody simulations and assist you in developing, optimizing, and automating your processes.
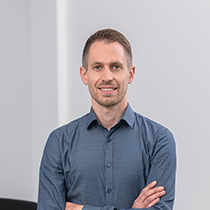
Dr.-Ing.
Manuel Eckstein
Head of Simulation + Predictive Maintenance
+49 931 49708-290
eckstein@woelfel.de
Contact form
Mechanical Engineering, TU Darmstadt
Dr.-Ing.
Mastering the variety of technical challenges together with our customers and with the Wölfel team through innovative solutions.
Nanostructure Technology (today's name: Quantum Engineering) at University of Würzburg
M.Sc.
Competence Network AI in SMEs "AI-HUB Northern Bavaria"
Real innovation requires not only knowledge, experience and creativity, but also a strong team and communication.
Because Wölfel is active across industries in the areas of expertise of vibrations, structural dynamics and acoustics, new challenges, customer requirements and knowledge transfers are constantly arising. This always opens up new use cases for the application of smart data-driven algorithms for us and our customers.
Mechanical Engineering, TU Darmstadt
Dr.-Ing.
Mastering the variety of technical challenges together with our customers and with the Wölfel team through innovative solutions.
Nanostructure Technology (today's name: Quantum Engineering) at University of Würzburg
M.Sc.
Competence Network AI in SMEs "AI-HUB Northern Bavaria"
Real innovation requires not only knowledge, experience and creativity, but also a strong team and communication.
Because Wölfel is active across industries in the areas of expertise of vibrations, structural dynamics and acoustics, new challenges, customer requirements and knowledge transfers are constantly arising. This always opens up new use cases for the application of smart data-driven algorithms for us and our customers.